What are the predictors of the party success of the Alternative fuer Deutschland (AfD) in the 2021 German federal elections?
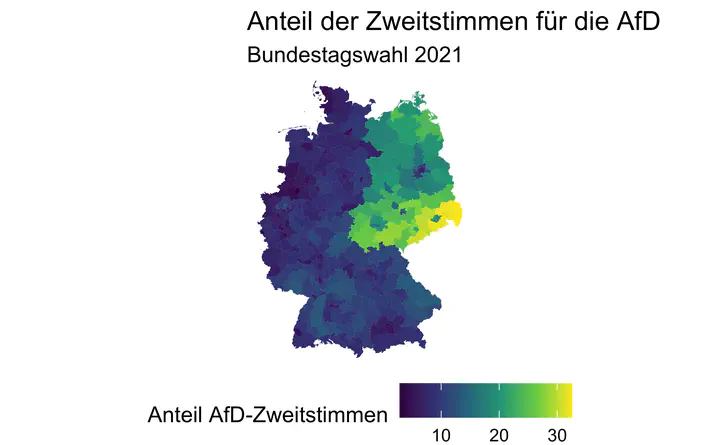
What factors fuel the AfD election success?
The party “Alternative fuer Deutschland” (AfD) is a right-wing to far-right political party in Germany. Founded in 2013, it that has managed to gain seats in all states parliaments as well as in the federal parliament within a short period of time. The AfD became the third-largest party in Germany after the 2017 federal elections. Such striking success is quite unique in German post-war politics. Consequently, AfD’s upsurge has sparked an intensive debate as to the why’s and hows of this success. Some explanations of AfD’s electoral success have been brought forward by scholars but also some “folk theories” circulate. In this study, we test some folk theories highlighting potential causes of AfD electoral success such as unemployment, migration rate, age, and east/west cultural differences. Our data are based on the German federal election results (2017), alongside with structural data on each German electoral district (n=299). Our analysis is novel insofar as a more rigorous Bayesian multilevel modeling is applied.
Methods: Bayesian multilevel regression
Sample. All data were obtained from the Bundeswahlleiter (2017). There were no missing values, and we confirm that the data were not transformed in any other way than reported here. z-Values were used as model inputs.
Hypotheses. Three factors were hypothesized to exert an effect on the AfD votes: a) unemployment rate (positive), b) foreigner rate (positive), and c) East Germany (positive). All effects are assumed linear.
Design. The study is based on a cross-sectional, observational design thereby precluding strong causal conclusions. Predictors were selected according to widely circulating folk theories of AfD success.
Analysis. Stan was used via the R packages rstan and rethinking (McElreath, 2017). For model estimation, Hamiltonian Markov Chain Monte Carlo (MCMC) with 2000 iterations, 2 chains, 1/2 burn-in time, was used. Information criteria (WAIC) served for model comparison. In an explorative matter, we included federal state as predictor and compared the model variations.
Results: Limited support for proposed model
Interestingly, neither immigration (foreigner) rate nor unemployment rate played an important role. East Germany proved to be a more impactful predictor. However, federal state came out as the strongest predictor of AfD voting success. In sum, the multi level model state combined state, foreigner rate, and unemployment rate turned out to be best in class (cf. Table 1, model m15_stan). Figure 6 compares the (absolute) prediction errors between all models tested. The trace plot of the best model supported convergence of the model (not depicted). Figure 7 shows the mass intervals for the main coefficients of the best model. A comparison between estimated and observed AfD votes per electoral district is shown in Figure 8.
Conclusion: Unknown role of State aspects
It can only be speculated as to why the state played a pivotal role in these models. A state can be seen as a bundle of shared cultural values, local socioeconomic factors, the impact of the aging society, or the influence of local politicians, to name a few. To be clear, the present model is simplistic. It remains unknown which (causally) relevant factors have been missed out. Sadly, the field lacks strong theories that explain the pathways of voting behavior, particularly for the rise of (right-wing) populist parties. Given the importance of political liberty, and in the light of the experiences (and horrors) of populism in the 20th century Europe, it remains a duty to shed light on the whys and hows of of societal and electoral will articulation.
Further work
Also check-out this related work on the possible impact of personality and voting for the AfD.